Exploring the Modern Data Analytics Stack: A Comprehensive Overview
- Tellius Inc
- May 6, 2023
- 2 min read
In the world of business, data is a valuable asset. To make informed decisions, organizations need to process, analyze, and interpret vast amounts of data in real-time. With the rise of big data and the increasing demand for data-driven insights, traditional data processing tools are no longer enough to keep up with the pace of innovation.
To address this challenge, a modern data analytics stack has emerged, consisting of a series of tools and technologies designed to handle the demands of big data analytics. In this blog, we will explore the various components of the modern data analytics stack and how they work together to provide insights that can drive business growth.
The first component of the modern data analytics stack is data storage. Traditional data storage systems like relational databases are not capable of handling the volume and velocity of data generated by modern business applications. To address this, organizations are turning to distributed storage systems like Hadoop and Apache Cassandra, which can scale horizontally to handle large datasets.
The next component of the Modern Data Analytics Stack is data processing. Tools like Apache Spark and Flink are used to process and analyze large datasets in real-time, enabling organizations to extract insights quickly and make informed decisions. These tools are designed to run on distributed systems, allowing them to process data in parallel and at scale.
Data visualization is another critical component of the modern data analytics stack. Tools like Tableau and PowerBI enable organizations to create interactive dashboards and reports that provide insights into their data. These tools allow businesses to quickly visualize trends, patterns, and relationships within their data, making it easier to understand and act on.
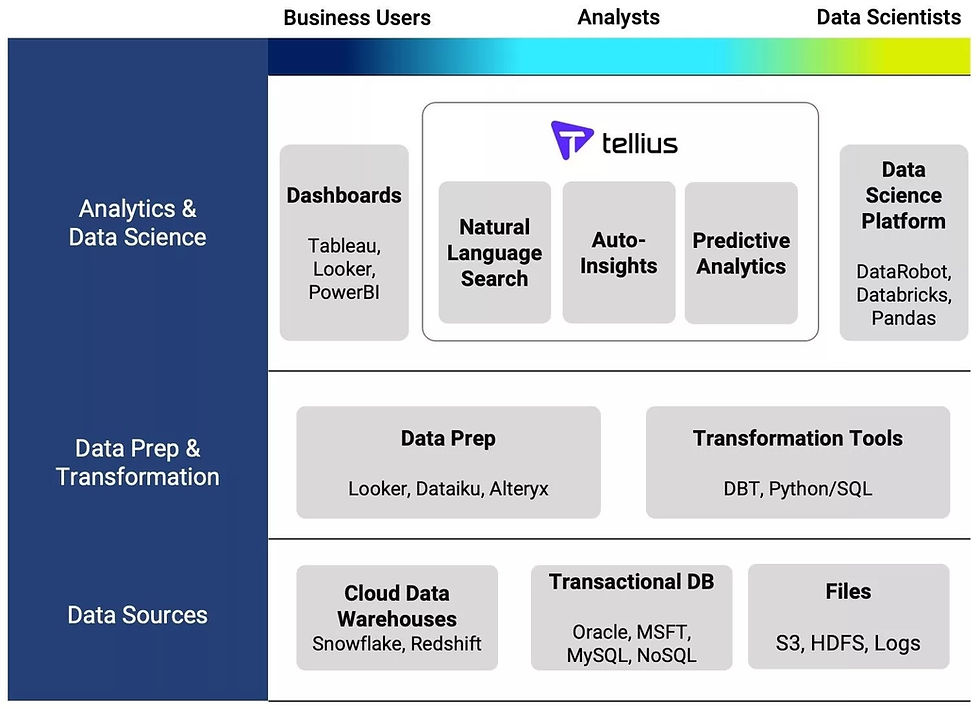
Machine learning is another key component of the modern data analytics stack. With the help of tools like TensorFlow and Scikit-Learn, organizations can build predictive models that can be used to make informed decisions based on historical data. These models can be used to identify trends and patterns, make predictions about future outcomes, and improve overall business performance.
Finally, data governance is a crucial component of the modern data analytics stack. As organizations generate and process more data, ensuring the security, privacy, and compliance of that data becomes increasingly important. Tools like Apache Atlas and Collibra provide data governance capabilities, allowing organizations to manage data access, track data lineage, and ensure compliance with regulatory requirements.
In conclusion, the modern data analytics stack is a powerful set of tools and technologies that enable organizations to process, analyze, and interpret vast amounts of data in real-time. By leveraging these tools, businesses can gain valuable insights into their data, identify opportunities for growth, and make informed decisions that drive business success.
The modern data stack is a comprehensive set of technologies and tools used to manage and analyze data in real-time. It combines cloud-based storage, data integration, transformation, and visualization to provide a streamlined approach to data management. This blog explores the components of the Modern Data Stack and its benefits in modern business operations.
Comments